- Fabio Puddu¹
- Annelie Johansson¹
- Aurélie Modat¹
- Jamie Scotcher¹
- Riccha Sethi¹
- Shirong Yu¹ ²
- Nick Harding¹
- Mark S. Hill¹
- Ermira Lleshi¹
- Casper Lumby¹
- Jean Teyssandier¹
- Michael Wilson¹ ³
- Robert Crawford¹
- Tom Charlesworth¹
- Robert J Osborne¹
- Shankar Balasubramanian¹ ⁴ ⁵
- Páidí Creed¹
1 biomodal Ltd, The Trinity Building, Chesterford Research Park, Cambridge, UK.
2 Current address: Tagomics Ltd, The Cori Building, Little Abington, Cambridge, UK.
3 Current address: Department of Astrophysical Sciences, Princeton University, New Jersey, US.
4 Cancer Research UK Cambridge Institute, University of Cambridge, Cambridge, UK.
5 Yusuf Hamied Department of Chemistry, University of Cambridge, Cambridge, UK.
A key challenge in genomics is the generation and integration of data across different modalities. This typically requires several assays to be performed, which is costly and time-consuming. Moreover, the subsequent integration of these data across assays is technically challenging. Here, we leverage an assay (Figure 1, [1]) that enables sequencing the complete genetic sequence and the DNA modifications, 5-methylocytosine (5mC) and 5-hydroxy-methylocytosine (5hmC), from low nanogram amounts of DNA, to provide 6-base genomic data.
Given 5mC and 5hmC play key roles in gene regulation and chromatin organisation, we aimed to explore how these multimodal data could further elucidate key biological processes and yield novel insight. To this end, we trained and evaluated a series of machine-learning models to predict gene expression, chromatin accessibility, and enhancer state from 6-base sequence data.

Figure 1 | duet multiomics solution evoC is a 6-base calling technology that reads all four canonical bases plus 5mC and 5hmC.
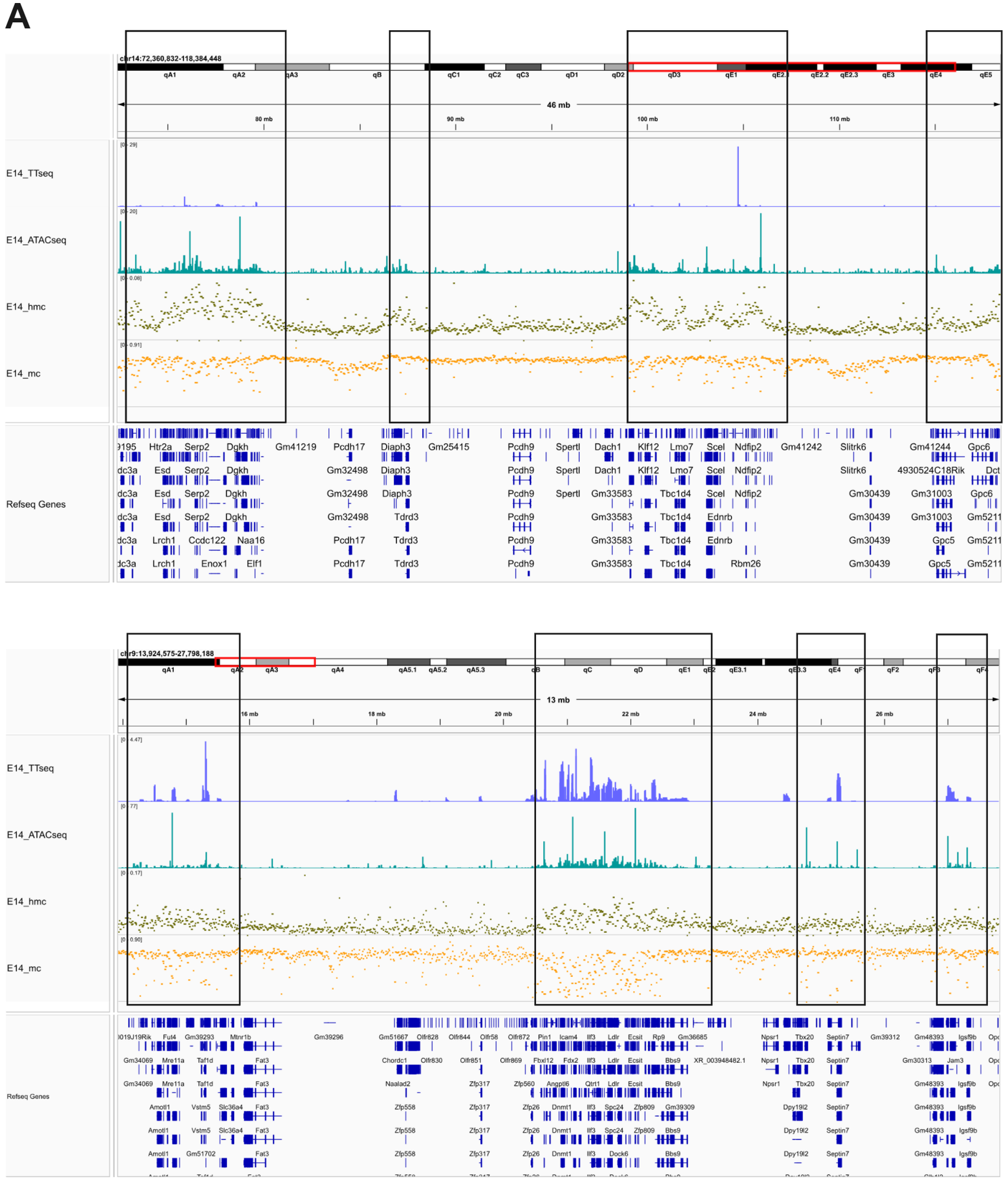
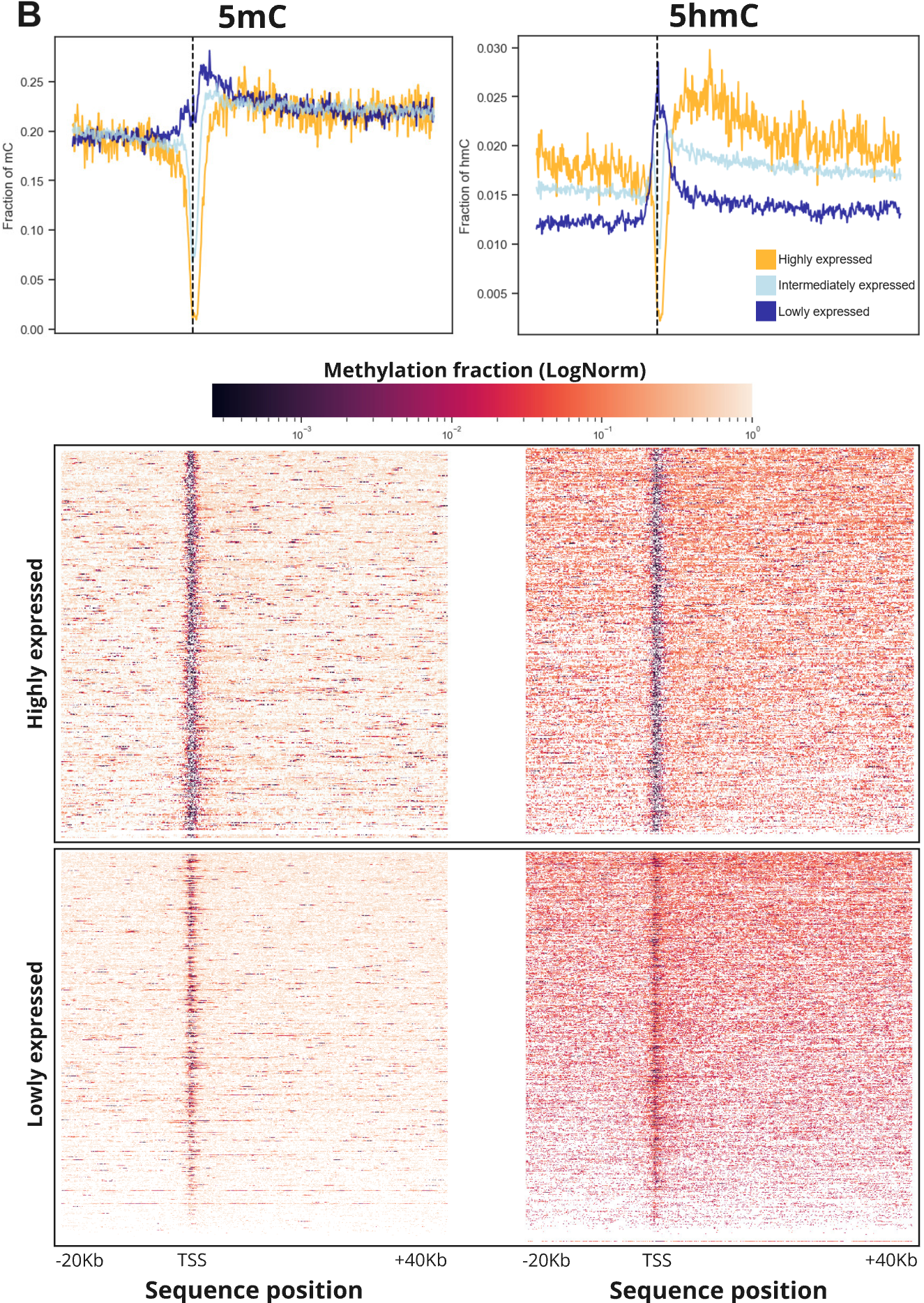
Figure 2
- IGV plot at two regions of the genome showing how 5mC and 5hmC modifications vary in open and closed chromatin as defined by ATAC-seq (teal bars), and how this pattern of variation also reflects newly synthesised RNA (TT-seq[2], blue bars). Cytosine modifications correlate with gene expression and these data are consistent with 5mC and 5hmC having opposing effects.
- Relationship between 5mC (left) and 5hmC (right) and categorical gene expression levels (high, intermediate low). The top panels show the mean methylation fractions across 60Kb regions around the TSS of genes in the E14 mESC cell line. The bottom panels represent similar information but show each individual gene rather than a combined summary. These plots highlight the different dynamics of 5mC and 5hmC around the TSS and into the gene body of genes with differing expression levels (quantified with nascent RNA sequencing data). In particular, this highlights how the 5hmC signal is able to clearly separate genes into high, intermediate and low expression states, whereas the 5mC signal is not clearly differentiated over these same classes.


Figure 3 | Using machine learning to relate 5hmC and 5mC patterns of variation to RNA sequencing (RNA-seq) and nascent RNA sequencing (newly synthesised RNA, TT-seq).
Here, we split the genome into a series of genomic regions (2kb upstream, 250bp around TSS, 5′ UTRs, first introns and exons, introns, exons, 3′ UTRs, and 5kb downstream), and computed the mean 5mC and 5hmC fraction from duet evoC measurements. These features (minus chr. 8), along with the number of CpGs and region length, were used to train an XGBoost regression model using published E14 mRNA data[2,3]. We used the withheld chr. 8. for testing. For RNA-seq data (A), we find good correlation (R²~0.75) between predicted and actual expression. For nascent RNA data (TT-seq, B), we found that the model was able to better predict expression with a slightly higher correlation of (R²~0.85). This finding highlights that 5mC and 5hmC constitute highly dynamic signals that capture aspects of nascent transcriptional states, yielding insight into real-time transcriptional dynamics.
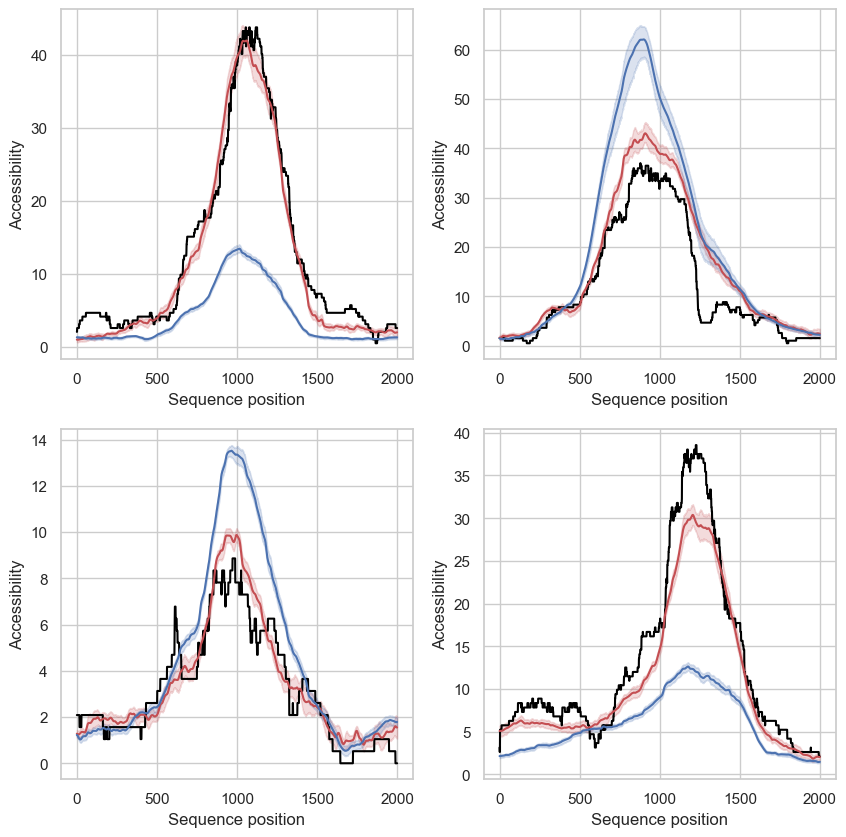
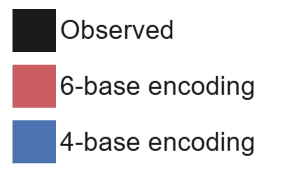
Figure 4 | Deep learning models to predict chromatin accessibility across sequences.
We trained dilated residual convolutional neural networks to predict base-resolved chromatin accessibility across 2000bp sequences, centered around TSS regions. These models consume a combined encoding of genomic bases (mm10 reference), 5mC and 5hmC (duet evoC) as input sequences and we used public ATACseq data from E14 mESC as the target output. During training we held out chromosomes 6 and 7 for validation and chromosomes 8 and 9 as the test set.
Globally, we find that models only trained using the genomic sequence (4-base encoding, R²=0.54) perform worse than models which also had access to 5mC and 5hmC as part of the inputs (6-base encoding, R²=0.62). Shown in the panels to the left are four example regions from the test dataset with ensembled predictions (10 random initialisations) from 4-base (blue) and 6-base (red) models.
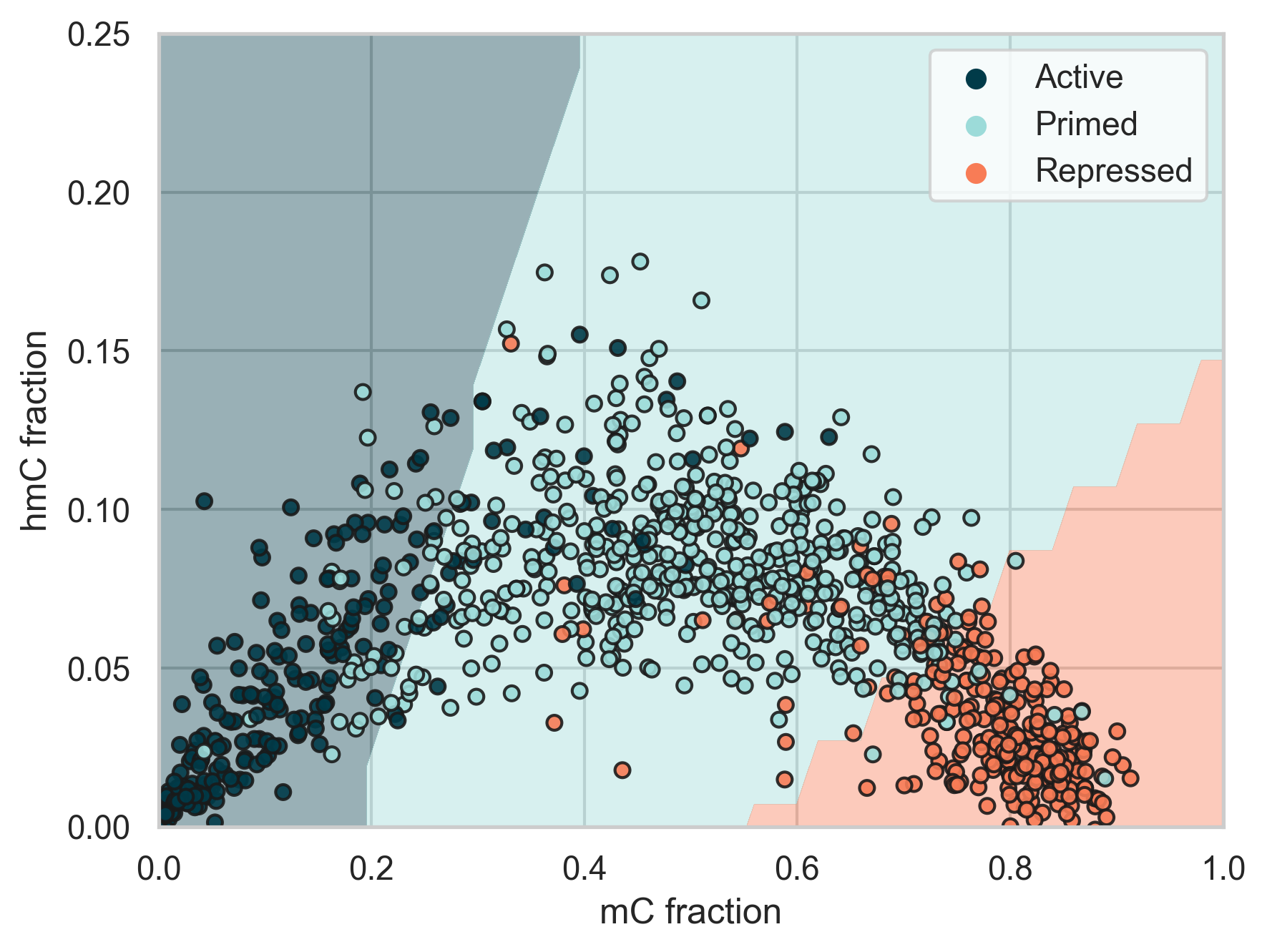
Enhancer states:
- Active: actively enhances gene expression (H3K4me1 & H3K27ac)
- Primed: is ready to activate gene expression (H3K4me1 but not H3K27ac)
- Repressed: Enhancers with none of the above marks, also known to be specific to various late stage tissues.
Figure 5 | Classification of enhancer states in the 5mC vs. 5hmC fraction space.
Enhancers are cis-acting regulatory regions that regulate cell-type specific gene expression programs. Context specific enhancer states are typically characterised by histone modifications in flanking nucleosomes [5]. Here we used the enhancer classes defined in [5] as labels and trained an SVM model to predict these labels using only 5mC and 5hmC fractions across each enhancer region.
We found that Active enhancers typically have low 5mC and 5hmC levels, primed enhancers typically have moderate 5mC and high 5hmC levels, and repressed enhancers typically have high 5mC and low 5hmC levels. Our trained model was able to predict enhancer states on 20% of the data that was held-out during training with 85.5% accuracy. In the plot the different background shades correspond to the SVM decision boundaries.
We have shown that the combination of resolved methylation and genomic data combined with machine-learning can generate accurate inference of gene expression (both steady-state and nascent), chromatin accessibility, and enhancer state, demonstrating the key role of 5mC and 5hmC in gene regulation. Moreover, there is the potential for a compounding effect whereby 6-base genomic assays not only yield direct data, but also the foundations for multiple other inferred modalities. Looking ahead, these approaches could enable novel insights into core biological processes and accelerate the speed of iteration for experimental projects, where one can yield multifaceted insights and even conduct pilot experiments in-silico using predictive models.
- Simultaneous sequencing of genetic and epigenetic bases in DNA, Füllgrabe and Gosal et al., Nature Biotechnology (2023). (duet multiomics solution technology paper)
- Acute depletion of the ARID1A subunit of SWI/SNF complexes reveals distinct pathways for activation and repression of transcription. Blümli S, et al. Cell Rep (2021).
- Cell Transcriptomics CRISPR-Activation Screen Identifies Epigenetic Regulators of the Zygotic Genome Activation Program. Alda-Catalinas C, et al. Cell Syst. (2020).
- Pioneer activity distinguishes activating from non-activating SOX2 binding sites. Maresca M, et al. EMBO J (2023).
- PRC2 Facilitates the Regulatory Topology Required for Poised Enhancer Function during Pluripotent Stem Cell Differentiation. Cruz-Molina S, et al. Cell Stem Cell (2017)